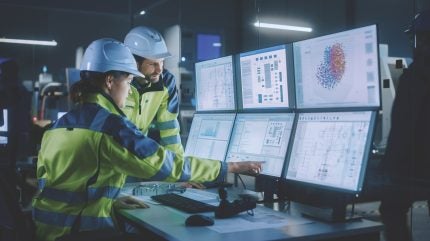
Artificial intelligence (AI) is an idea that is pervasive at the moment, both talked up as the solution to many current problems and talked down as a risky technology that could take control out of human users’ hands. Nicolas Prettejohn, Head of AI, UK & Nordics at Palantir, summarises it as “software that helps companies tackle their most critical problems”.
Prettejohn explains that AI is not about doing simple things more quickly, as has often been the rationale for types of automation, whether hardware or software. Instead, it is about giving people the ability to do difficult things.
He gives a simple example of a task that is more complex than it seems: asking questions in unstructured language about consenting, in an international company where documents are in different languages and refer to different permitting regimes. He says, “It’s those things that are most useful. Civil society will talk about AI not being used in critical circumstances – but that’s where all the benefit is. We need to find a way of thinking about risk and mitigation.”
It is true there is a risk that the AI will give the ‘wrong’ answer to an unexpected question. An AI issue that is often highlighted is the risk of so-called ‘hallucination’. Prettejohn compares this with the experience of working with junior employees. They may also come up with outliers, he says, “but you manage that within the organisation. You have oversight, checks and balances, and people checking each other’s work. Of course, there are some risks that can never be fully eradicated and there is where companies use insurance.”
Prettejohn says most people have proxys for AI – refering to the control rooms in nuclear power plants, which have evolved over the decades from hard-wired ‘red light’ alarms to screens providing both detailed information that can be interrogated and recommended actions. But, he suggests the AI is more of a colleague. He explains, “If everyone had an assistant you would be able to debate ideas all the time, especially if they had your own knowledge base and operations experience – it would be a collaboration. The big objective of some of our most successful deployments has been that the end user sees an interesting perspective they had not thought about.”
“With an AI ‘assist’, a language model can also access enterprise knowledge and maybe explore something from the data store that the user had not appreciated.” Because the AI has the full sum of all the knowledge “You actually uplift your operators,” he says.
Prettejohn speaks from Palantir’s experience of using AI in the health industry. He stresses that the first aim has to be to improve quality, because that gets buy-in from the users. He says, “We always think about AI in terms of efficiency gains, but what we learned with hospitals is that you don’t get efficiency gains by using AI. People care about their work and they will never adopt a technology that makes them quicker if it doesn’t deliver better care. Never.” Focusing on delivering better care might include, for example, producing clearer, more precise clinical notes and scheduling faster or more appropriate appointments. “If the AI delivers a better outcome, that makes them feel they can rely on it and use it. Then you get the efficiency gain ‘for free’.” He summarises: “By encoding expertise you scale it up.”
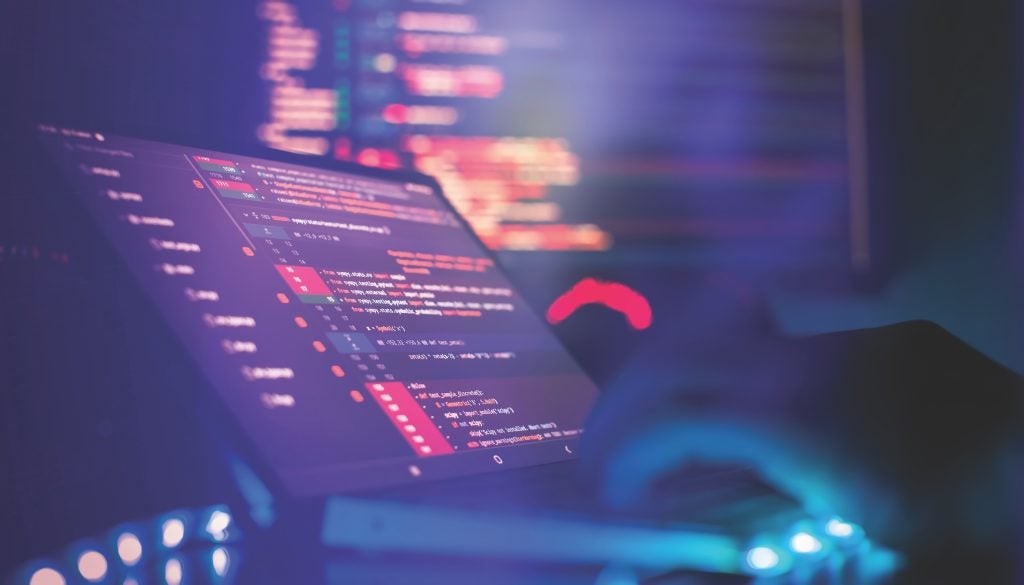
Ways of addressing the world
Prettejohn expands on some of the limitations of existing systems. “The main thing we try to do is get people away from thinking in the way their ‘source systems’ see the world.” He says source systems (which may be software programmes used to run or maintain an asset, or any type of information store) “have – almost by design – an idea of looking through a keyhole at the world.” For example, one system might show how much power is being delivered through a wire, but maintenance logs are in another system that does not ‘understand’ what is going on in the wire.
Prettejohn imagines a user who has to make critical decisions about what is going on from this variety of systems, and wants information in a form that can be used easily: “You are looking for a view of the system that tells you ‘that wire is down, in that place, it is affecting this many customers, of which four users have medical need for power.’” At that point, he says, you are not worrying about whether the information is in a column in that database or a row in another database. “We try to build systems that mirror how people actually solve problems and the way they represent data when they solve problems. Facts and information and semantics. That requires a different sort of system,” which connects all those old and new representations of what is going on, does it in real time and presents it as an aid.
What does this mean in practice? With an issue like the failed cable discussed above, typically there would be action points to fix the problem, but the details remain in the various software systems and in various different forms depending on the data source. He explains “We start to build these [details] into the software. But then you can start to run those actions against a digital twin. Your semantic model would ask ‘if we did this what would happen, what if we did this’? Finally, you get a schedule for action” that is backed with data, experience, technical detail etc, which the AI system can ‘write back’ to systems like engineering and maintenance, adding jobs to the queue, changing someone’s job or carrying out other actions. Most importantly, the user can ask unstructured questions using normal syntax, not coding.
Prettejohn says, “Having that deep information in the system means we are not a silo, not a dashboard, not an exhaust port of useful information. This is a control room for running a business that looks like how we want to talk about the business and understands how we want to think about the business.” He adds, “It is a far more effective control room than what was previously happening where someone would be logged into eight screens with a notebook trying to run the business. Now they can have it on one single panel” using syntax familiar to the user.
Prettejohn adds that the system will develop “an understanding of semantics and deep in that network they start to understand the relationship between entities, what the relationship is and the intent of what we are communicating versus the syntax”.
Safety cases
Palantir is exploring using its AI to help civil nuclear operators write safety cases much more quickly. The company’s engineers have been looking at this opportunity. They say the nuclear industry now has new needs in writing safety cases, for a number of reasons.
First is the scale of investment going in, with many new designs and new projects under way. “The volume of safety cases required has gone through the roof… We have a situation where safety cases need to be produced at scale very quickly” says Prettejohn. They compare that with the safety case writers available now: “They have generally been in organisations for a very long time and people underestimate the niche of that skills set – it is a very specialised skill. As we look at attrition and retirement you have a rise in demand for safety cases, with a decline in the number of people able to produce them. That is the crux of the problem. Something has to change.”
The company has been looking at how it can speed up the process. Prettejohn explains: “When we think about nuclear safety cases, they are not simple. Each is normally hundreds of pages and there are typically hundreds of documents in a safety case portfolio. This is a lot of paperwork.” The safety case for a single building can fill “lockers and lockers” – and that is a real image, because in some cases it involves original paperwork that is not captured digitally anywhere.
The company is looking at how safety cases can be created more quickly. One opportunity is to be able to reference old safety cases and pass appropriate parts forward to new safety cases. Palantir’s software uses embedding models to quickly scan text and find parts that have to be referenced in a future safety case. This is not like searching for a keyword, Prettejohn explains: “It is the context that is interesting, not the keyword, so it is much more effective to do a semantic search than to search for key words. You find all the different applications where the word is used and see that some are useful.”
This is about saving time, rather than cost. “Look at what goes in to building a nuclear reactor and the cost and saving head count is not the thing that worries you… But being able to reference the old and bring it into the new is important.”
Another aim is document validation and curation. “Safety cases are hundreds of documents and thousands of pages and you need to be able to reference documents to one another.” Making changes in one document, making sure they are made in all the other documents and ensuring they are up to date in them all – is “a really difficult thing to do.” Prettejohn says, “These documents are not just written by one person. Even if it is drafted by one person there are revisions and revisions, written by different stakeholders who are required to go through a safety case before it is approved. So understanding when a safety case has been revised is really important, and so is looking through two variants of a safety case to look at what has been revised and why. Then you want to be able to apply those learnings to any future safety cases you are generating, to accelerate that process.”
He takes the opportunity a step further, in the context of hundreds of safety cases being written. Talking about a national programme such as the UK’s, he asks, “If you could bring them into a central platform and use those features, are you able to generate safety case documentation from scratch?
“You always need humans in the loop and humans should always be actively employed in writing safety cases, but there is a lot of safety case content that has nothing to do with the specifics of what is being approved. There is lots of generic stuff that has to go in and lots of risks that are relevant through lots of different safety cases.”
Taking all the context of previous safety cases, you would ask AI to make a new safety case that looks broadly like something existing, and pull in all the relevant content. That way, “You don’t have to start from scratch, you have a scaffold… and we all know that the hardest part of writing documents is starting. Being able to mark someone else’s homework is a lot easier.”
Finally, he says that “safety cases are not just done by one person and signed off, they go through lots of different stakeholders.” With a large number being done in parallel, “at any one time you want some kind of system that will be able to track where every document is at any moment in time.” What stakeholders have seen it? What parts have been reviewed? What are the comments that have been made? What adaptations have been made? Where is the audit? Who has approved what, and when?
This becomes very important when considering workflow during construction. Large sites like nuclear plants have thousands of people and complex information that takes days or weeks to review. Any large capital project can build up a stack of ‘to approve’ documents and undocumented work in progress. What is more, doing something differently – and better – requires safety case approval. But people don’t have the tools to compare and contrast sections on several documents and there is no simple way to say ‘these are the points you have to look at’. However, AI can answer an unstructured question such as ‘can I excavate here?’ by interrogating all the documents that relate to that square metre of land.
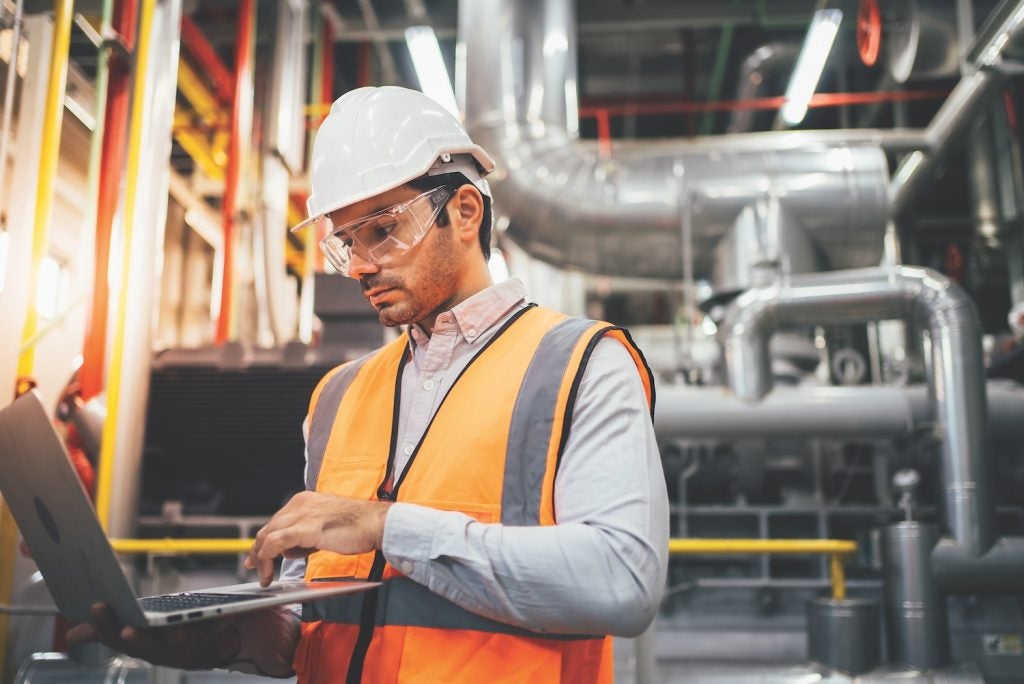
Reasoning not regulation
In more general terms, Prettejohn says, “What we are trying to teach the AI isn’t regulation. We are trying to teach reasoning.”
He uses an analogy from a much simpler process – checking invoice details against contract terms. “We don’t train it on every contract because that’s not verifiable. Instead, we teach it how to do it. This is all logic relationships.”
Translating that to a safety case example, “you would have all the regs, split out clause by clause. If you have any case history you would bring that in as another object type, you can bring in lawsuits – here is where regulators have taken action.” He says, “The point is to use the AI for what it is good at, which is essentially pretending to reason about things, and not use it for what it is bad at, which is remembering facts.”
He goes back to his analogy of AI as a colleague: “It is never tired and if you don’t like the outcome you can get it to do it again, so you have the opportunity to revise as often as you like.”
Prettejohn also talks about the ‘tribal knowledge’ that arises in a department and accessing the expertise and experience locked away in people’s heads. “New people coming in have to spend many years building up that knowledge,” he says, “It is inaccessible and without years of shadowing them you won’t be as effective as them, because the knowledge is locked away in their head – however good they are at telling someone else, that will always be imperfect.” That affects the ability of the organisation to be agile, or to bring on new members of the team to be as good as the individuals who have been around a long time. “With that tribal knowledge encoded I could turn up tomorrow and although I know zero about a nuclear safety case, if I had something like this with all that logic built in already, I wouldn’t be completely ineffective for three years trying to learn it. You could be at least semi effective after a couple of weeks or months.
“When you look at it in those terms it is industry-changing.”